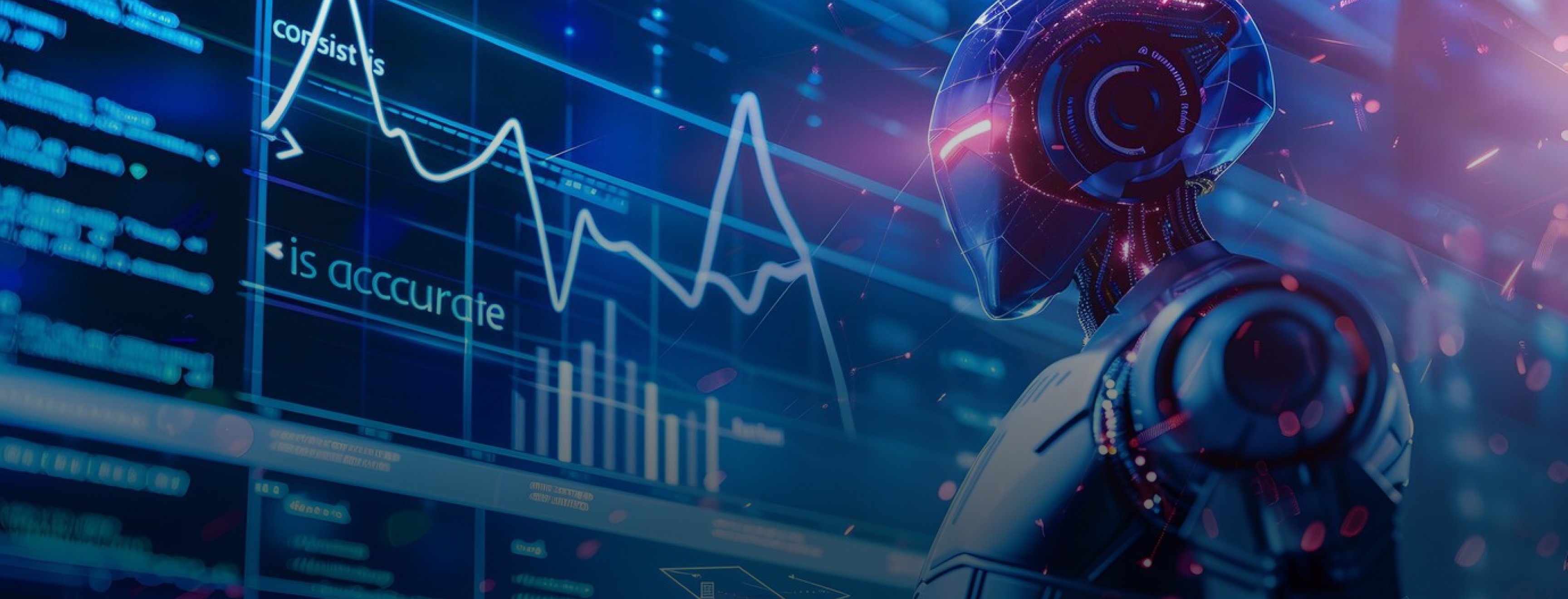
Big Data Analytics Services
Thareja unlocks the power of Big Data to help clients compete more effectively in today’s digital world.
Section Navigation
Thareja Big Data Analytics Services
In today’s data-driven world, the ability to harness and analyze vast amounts of information is critical for making informed business decisions. Thareja offers a comprehensive suite of Big Data Analytics services that empower organizations to transform raw data into actionable insights, drive innovation, and maintain a competitive edge. Our tailored solutions are designed to address the unique challenges of managing and analyzing large datasets, ensuring that businesses can extract meaningful value from their data while maintaining efficiency and scalability.
Big Data Strategy
A solid big data strategy is the foundation for successful data-driven transformation. Thareja works closely with clients to develop customized strategies that align with their business objectives. We help organizations identify key data sources, define analytics goals, and establish a roadmap for data management and analytics implementation. Whether you are just starting your big data journey or looking to optimize existing processes, our strategy consulting services ensure that your data initiatives are aligned with your long-term vision.
Data Management
Efficient data management is crucial for leveraging big data. Thareja provides end-to-end data management services that ensure the proper collection, storage, organization, and governance of your data. Our solutions include data integration, data quality management, metadata management, and data lifecycle management. By implementing best practices in data governance and security, we help businesses ensure that their data is accurate, accessible, and compliant with industry regulations.
Cloud & Infrastructure Services
Big data often requires scalable and flexible infrastructure to process large volumes of data efficiently. Thareja offers cloud and infrastructure services to support your big data initiatives. Our cloud solutions enable businesses to store and process massive datasets on platforms such as AWS, Azure, and Google Cloud, providing the elasticity and cost-effectiveness needed to manage big data workloads. We also offer on-premises and hybrid cloud solutions for organizations that require customized infrastructure setups. Our infrastructure services ensure that your big data solutions are scalable, secure, and optimized for performance.
AI and Machine Learning
Unlock the full potential of your data with AI and machine learning capabilities. Thareja designs and implements advanced AI-driven solutions that allow businesses to derive deeper insights from their data. Our machine learning models can help with predictive analytics, anomaly detection, sentiment analysis, and more. By integrating AI into your big data analytics strategy, you can automate decision-making, enhance personalization, and uncover trends that drive innovation and growth.
Analytics and Insights
Data is only valuable when it can be transformed into actionable insights. Thareja analytics services offer a comprehensive approach to analyzing structured and unstructured data. We leverage advanced analytics techniques such as predictive modeling, prescriptive analytics, and real-time analytics to help businesses make data-driven decisions. Our team of data scientists and analysts work with you to uncover insights that improve business operations, optimize customer experiences, and identify new market opportunities.
Reporting and Visualization
Effective decision-making requires clear and actionable data representation. Thareja provides reporting and data visualization services that turn complex datasets into intuitive and interactive dashboards, reports, and visualizations. Our solutions use leading platforms such as Tableau, Power BI, and custom visualization tools to present data in a way that is easy to understand and use. Whether you need to monitor KPIs, track performance metrics, or visualize trends, our reporting and visualization services help you make sense of your data in real-time.
Data Governance and Compliance
As data privacy regulations continue to evolve, maintaining data governance and compliance is essential. Thareja data governance services ensure that your data management processes are secure, compliant, and auditable. We help businesses establish data governance frameworks that align with global standards such as GDPR, HIPAA, and industry-specific regulations. Our solutions focus on protecting sensitive data, ensuring data integrity, and maintaining transparency across the organization.
Industry-Specific Big Data Solutions
Different industries have unique data challenges and opportunities. Thareja offers industry-specific big data solutions tailored to the needs of sectors such as healthcare, finance, retail, manufacturing, and government. Whether it's managing patient data in compliance with healthcare regulations, analyzing customer behavior in retail, or optimizing supply chains in manufacturing, our big data solutions are designed to deliver results that matter to your business.
Why Thareja
With decades of experience and a commitment to innovation, Thareja is a trusted partner for organizations looking to unlock the full potential of their data. Our holistic approach to big data analytics covers everything from strategy development to data management, cloud services, AI integration, and data visualization. Whether you're looking to improve decision-making, optimize operations, or gain a competitive edge, Thareja Big Data Analytics services provide the tools and expertise to turn data into business value.
For more information about Thareja Big Data Analytics services, Contact Us.
The Role of Big Data in Digital Transformation
Data is one of the most critical aspects of any digital transformation strategy. No investment in digital transformation will produce a return on investment if there is insufficient or unreliable data to support digital services or processes. Beyond data availability and quality, is the issue of accessibility and integration. Data which sits in a silo may hold tremendous promise but may be practically worthless if it cannot be accessed easily and integrated with other data sources to create a deeper understanding of a process or opportunity. As such, every digital transformation strategy should include a significant focus on data.
Does the data exist?
An important issue to consider when planning a digital transformation should be consideration of whether the data needed actually exists. To answer this question, it is often best to start at the end. By understanding the types of analyses and reports that will be required, organizations can work backwards to determine the types of data that are needed to support the desired analysis.
If the data exists, it is important to consider the following:
- Is the data of good quality and reliable?
- Can the quality be improved?
- Where is the data housed?
- Is the data usable and accessible to the extent needed to support the analysis?
Conversely, if the data does not exist, can the data be generated? If the data can be generated, the same four points above should be considered for the newly generated data. If the data cannot be generated, digital transformation does not have to be abandoned. There may be ways that a missing dataset can be replaced with a close approximation created through data modelling of a related dataset, or based on the market experience of subject matter experts. migration moves data, applications, and computational workloads from physical servers to the cloud.
The importance of data management
Creating a data management strategy is a critical aspect of any digital transformation initiative. Data management includes a series of processes to help improve the quality of data, ensure it can accessed to the extent needed for proper analysis, and to create integrations with other datasets which are needed to support planned analyses.
Improving Data Quality. The first step in data management is improving data quality. This assessment should include consideration of the relevance, accuracy, format, and completeness of the data. To address issues that might be identified here, data cleaning and manipulation is often needed. It is critical that when data manipulation is required that it does not introduce bias into the dataset, and that all data manipulation is done objectively and consistently. Artificial Intelligence (AI) can be an excellent tool for improving the data quality of large datasets. A well planned AI can quickly and reliably perform data manipulation on vast amounts of data making datasets which previously impractical to use, a valuable resource for organizations.
Ensuring Accessibility. A reality of legacy technologies and systems are data silos. Many organizations continue to use old software and systems that are no longer supported by the original software vendor and lack the integration APIs needed to be able to easily work with the data in conjunction with other data sets.
The best way to remove the data accessibility barriers created by legacy systems is to replace or upgrade the them. Where budgets allow, a modern cloud-native replacement for a problematic legacy system could not only help resolve data-related challenges, but also provide many operational advantages to the organization. Because system upgrades or replacements are often not feasible within available budgets, data integrations must sometimes be relied upon to create work-arounds to improve data accessibility.
Integrating datasets. The majority of digital transformations that fail, do so because of problems with the data integration. Creating an unified data layer is a key tactic for eliminating data silos, data fragmentation and ensuring data quality. A unified data layer is an architecture that, when used in conjunction with a data strategy, integrates disparate datasets in a disciplines way to create the holistic views needed to power digital transformation. A strong digital transformation vendor can guide organizations on creating an unified data layer that can reliability overcomes data integration and quality issues.
The benefits of digital transformation can be many. Those benefits are only possible if the digital transformation strategy is supported by high quality data. For more information about Thareja digital transformation and data management services, contact us.
For more information about Thareja Big Data Analytics Solutions, Contact Us.
Best Practices for Developing a Big Data Analytics Strategy
Many companies have embarked on a big data strategy to better understand their customers and optimize management of their operations. The path to big data success is often now a smooth one and often fraught with challenges like poor data quality, resistance to adopting new data sources, and getting timely access to insights and other issues. Fortunately, there are some well-established best practices organizations can follow to help make their big data strategy a success. Here are a few approaches to consider:
Start at the end - Intuitively, many companies start their big data analytics journey with exploratory analytics using the data they have available. This process can be used to identify patterns and trends but often does not progress to a point where it supports better business decision-making. For this reason, it is important to start at the end. Starting the big data journey by considering the decision points that are most important to your business allows you to work backwards to identify the types of data that are most relevant to this chosen decision-making process. This approach helps you proceed with greater confidence that your big data journey will produce meaningful benefits for your business.
Build an analytics culture - Great business intelligence and insights are only valuable if they are used. Employees’ comfort with older processes and procedures can be a significant barrier to implementing a big data strategy. A key aspect of any big data strategy should be building a big data culture. Create an environment where the people working with the data understand why the data is important, how it will be used, and the benefit it will produce if used properly. With a positive big data culture, you will be able to set yourself up for big data success.
Re-engineer data systems - As the name suggests, big data involves working with large volumes of data. Existing systems are often unable to cope with the demands for computing power and storage that big data requires. Extracting value from data typically requires analysis, visualization, and collaboration tools that need to be accessible in real time. Companies need to re-engineer their data systems for analytics by using distributed architectures and enabling flexible access to data sources.
Focus on useful data islands - Older enterprise systems often produce data islands, discreet sets of data that were not connected to other data sets. Data sets used in manufacturing may be separate from those produced by digital marketing or supply chain processes. After recognizing these data islands, using them effectively may simply be a data visualization exercise which can provide directional insights to inform other business functions where a correlation is found. Where data islands relate to various steps in a larger process, it may be worthwhile to consider integrating the data islands to create a larger data set that provides insights and visibility into the overall process as opposed to the just the individual components represented by each data island.
Emphasize business value - Business value is one of the most important aspects of big data analytics. Prioritizing big data investments and efforts based on the revenue and operational gains they can produce for the business helps ensure the success of your big data strategy. Big data, in isolation, produces insights. Big data that is analyzed to inform important business challenges or opportunities produces business value.
Iterate often - Building your big data analytics model is an iterative process with each version representing a refinement of previous versions. The initial model provides preliminary results which help validate the overall initiative and serve as a basis for beginning the refinement process. Iterating often helps build a solid foundation for your analysis, improving its accuracy and reliability over time.
Standardize your approach - To ensure the ongoing reliability of data feeding into your big data analytics program, and the analyses being performed, standardization is critical. The best way to do this is to set up a repeatable process for ingesting, cleaning, storing, analyzing, and presenting data. This approach will not only improve the reliability of your big data analytics program, but it will also allow you to cut down on time spent on each step. The discipline that comes from standardized processes can also minimize data and analytical errors at every step of your analytics process.
Continually test your big data assumptions - It is important to approach your big data analytics program with an open mind. Long held business assumptions or processes do not always hold true when examined through the lens of new data sources or algorithms. Testing is key. Testing new assumptions and algorithms against older ones allows you to objectively determine which approach produces greater value for the business.
Conclusion:
The key to successful big data analytics programs is a systematic and consistent approach. For information on how Thareja can help you make your big data analytics strategy a success.
For more information about Thareja Big Data Analytics services, Contact Us.
Data Quality Best Practices for AI
Experts agree Artificial Intelligence (AI) and Machine Learning will revolutionize how we live and conduct business. The key to realizing this vision is data. Data is the foundation for building robust and functional AI solutions.
Because of the important role data plays, the quality of data is critical. Data must be accurate, relevant, complete, timely, and consistent for it to be of value for AI purposes.
The following are eight steps to ensuring high quality data for AI solutions:
Quality control of data. As data is often produced by outside sources or applications, organizations may have limited control of the data generation process. As such, thorough quality assurance may be the best option for ensuring data quality. Because of the volume of data included in AI datasets, the process to ensure data integrity should be automated so resources can be focussed on addressing issues as opposed to identifying them.
Data governance. It is important to establish data management procedures that recognize and proactively address issues which can introduce errors into the data. The scope of data governance should include the collection, storage and use of data and pay close attention to prevent user and applications from unintentionally altering the data by overwriting it or introducing duplicate information.
Data accuracy. Another important aspect of ensuring quality data for AI is ensuring the data accurately explains the behaviors or activities that it is intended to inform. The approach taken should be scenario-based and incorporate the operational scenarios envisioned for the AI.
Data Labelling. Data labels not only allow an AI to understand the data it is consuming, it is also invaluable when investigating data anomalies. Datasets with proper meta data facilitate easier investigation of data problems allowing them to be resolved more quickly.
Data Traceability. Another important requirement for resolving data anomalies is access to source data. Being able to track data to its source records may sometimes be the only way to reliably resolve issues identified in data sets.
Noisy Data. Noisy data is data that cannot be understood by machines. It is often meaningless or corrupted data. Noisy data often causes machine learning or artificial intelligence algorithms to miss data patterns thereby impairing their ability to learn tasks. Data cleansing process need to identify and remove noise.
Data Augmentation. Some AI models require a higher volume of data to perform optimally. It may be necessary to supplement data sets with additional data to properly support AI algorithms. This can sometimes become an issue when dealing with noisy data.
Feature Engineering. When the dataset is not able to inform the AI algorithm, feature engineering can be used to supplement the data with additional variables or by creating features in the data to overcome the shortfall. This is best undertaken by engaging subject matter experts.
A comprehensive data quality strategy is critical to increase accuracy, reduce the cost, and speed AI implementation.
For more information about Thareja Big Data Analytics services, Contact Us.
About this gig
About 90% of the businesses I work with, require a custom AI agent (instead of a pre-designed one). Usually businesses reach out when they have exhausted the capabilities of all the existing no-code Agent builders (which are very limited). An AI agent for a business is at the end of the day, enterprise software and needs to be built and operated as such. I'll provide everything you and your team needs to run the agent in house or I'll suggest the best tools and services for you to run it on third party infrastructure.
Compare packages
Basic
Kid A
I will create a basic custom AI agent that performs a single task for you
Standard
Kid B
I will create a custom AI agent that performs up to 3 different tasks for you
Premium
Kid C
I will create a custom AI agent that performs up to 3 different tasks and connects to up to 5 tools.
FAQ
What are your deliverables?
I will deliver all source code, config files and documentation for you to run the agent.
Can you run/operate the agent for us?
Yes, I do this for a lot of companies. Happy to do it for yours.
Why is this so expensive?
It is actually, cheaper if you make numbers. You might end up paying same to an intern to do half of what the agent will do for you.
This service is offered by a Pro freelancer
- Hand-picked freelancer
- High-quality work
- Experienced with businesses
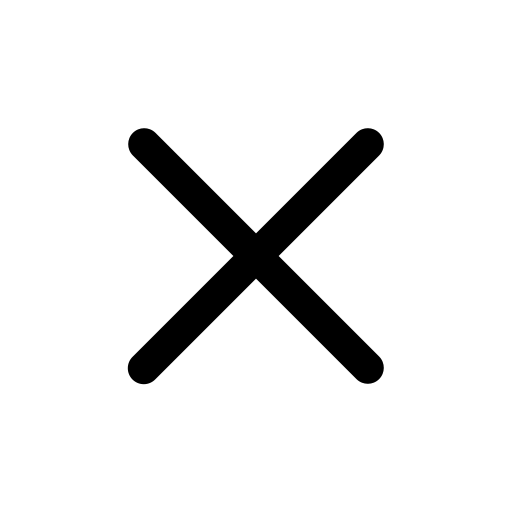
- Basic
- Standard
- Premium
Kid A
$8,000I will create a basic custom AI agent that performs a single task for you
-
Functional AI Agent
-
Agent Custom Development
-
Source code
-
Setup file
-
Detailed code comments
Kid B
$12,000I will create a custom AI agent that performs up to 3 different tasks for you
-
Functional AI Agent
-
Agent Custom Development
-
Source code
-
Setup file
-
Detailed code comments
Kid C
$18,000I will create a custom AI agent that performs up to 3 different tasks and connects to up to 5 tools.
-
Functional AI Agent
-
Agent Custom Development
-
Source code
-
Setup file
-
Detailed code comments
Global Operations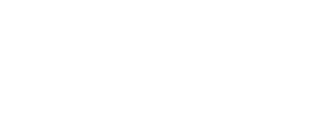
Our mission is to empower professionals to thrive and deliver exceptional work—anytime, anywhere.
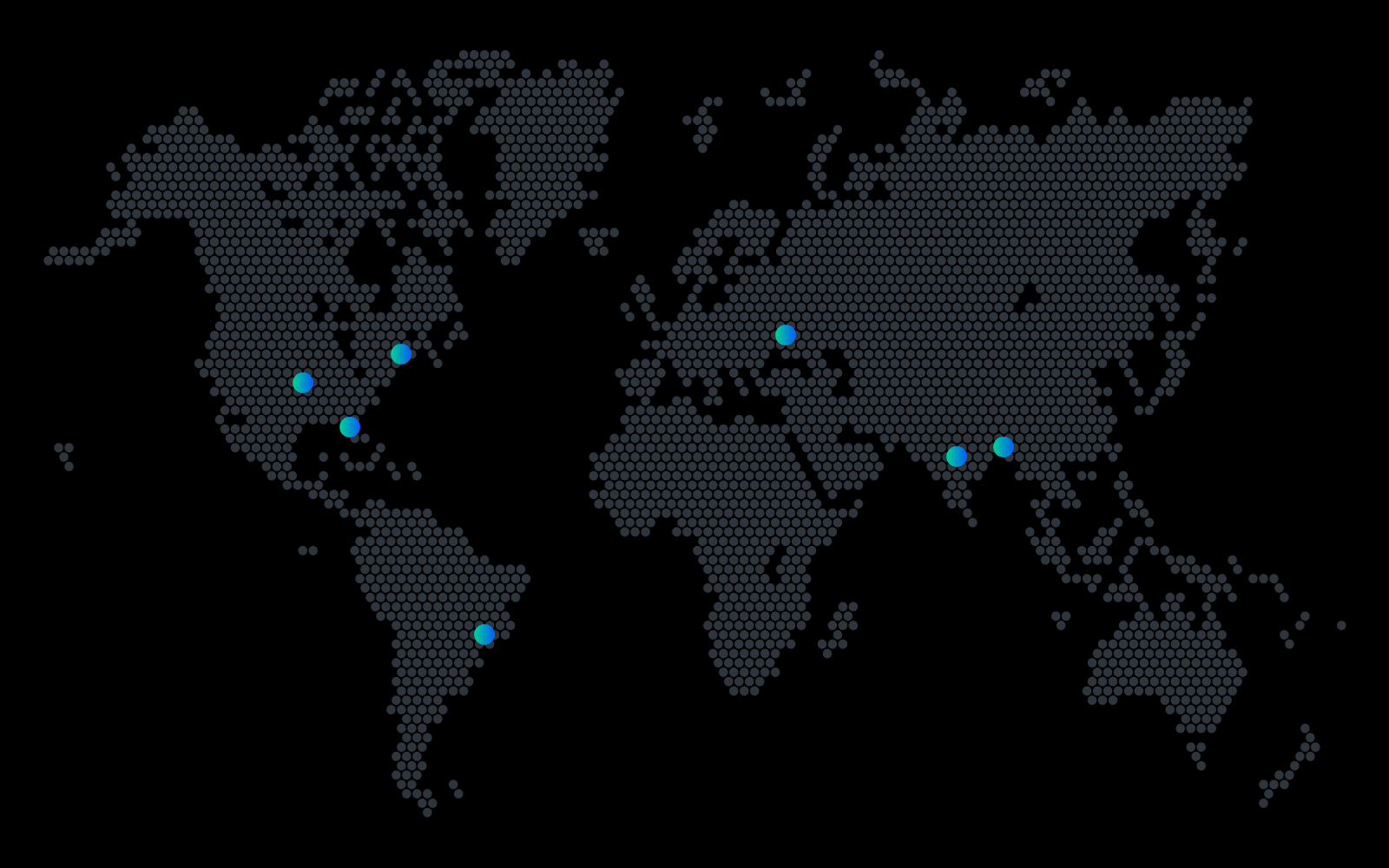
4.9 Stars
Google Reviews
Everything we do, we do it for our users. We care about product quality, attention to detail, and making a positive impact on as many people as we can.
600K+
Downloads
Hundreds of thousands of users around the world downloaded our 9+ apps.
Thareja AI has been used to deliver exceptional services for top brands around the world.
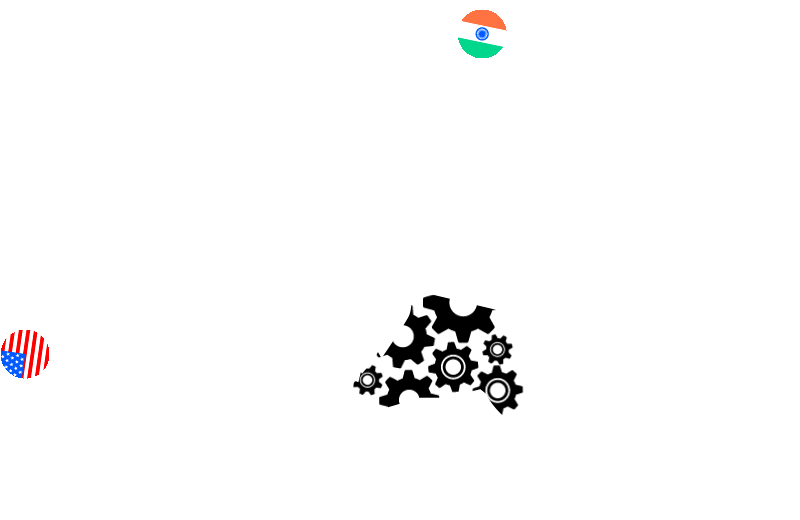